ANALISIS PERBANDINGAN KORELASI SPEARMAN DAN MAXIMAL INFORMATION COEFFICIENT DALAM SELEKSI FITUR WEBSITE PHISHING MENGGUNAKAN ALGORITMA MACHINE LEARNING
Sari
aan yang menipu maupun secara teknis untuk mencuri data identitas pribadi konsumen dan kredensial akun keuangan. Phishing dirancang untuk mengarahkan konsumen ke website phishing yang menipu penerima untuk membocorkan data keuangan seperti nama pengguna dan kata sandi. Dalam dataset phishing, terdapat fitur-fitur yang bisa mengkategorikan apakah sebuah website adalah website phishing atau bukan. Tujuan dari penelitian ini adalah untuk membandingkan hasil seleksi fitur-fitur yang ada dengan menggunakan dua metode yaitu metode gabungan Maximal Information coefficient dan Total Information Coefficient dengan metode korelasi Spearman. Hasil seleksi diuji dengan lima algoritma machine learning yaitu, Logistic Regression, Naïve Bayes, J48, AdaBoost MI dan Random Forest. Hasil dari penelitian ini adalah metode gabungan Maximal Information coefficent dan Total Information Coefficient memiliki nilai akurasi 97.25 % dengan menggunakan Random Forest mengungguli metode korelasi Spearman dengan nilai akurasi 95,33%.
Kata Kunci
Teks Lengkap:
PDFReferensi
P. Yang, G. Zhao, and P. Zeng, “Phishing website detection based on multidimensional features driven by deep learning,” IEEE Access, vol. 7, pp. 15196–15209, 2019, doi: 10.1109/ACCESS.2019.2892066.
“Phishing Activity Trends Report Q1 2020,” 2020. https://docs.apwg.org/reports/apwg_trends_report_q1_2020.pdf.
H. Y. A. Abutair and A. Belghith, “Using Case-Based Reasoning for Phishing Detection,” Procedia Comput. Sci., vol. 109, pp. 281–288, 2017, doi: 10.1016/j.procs.2017.05.352.
C. L. Tan, K. L. Chiew, K. S. Wong, and S. N. Sze, “PhishWHO: Phishing webpage detection via identity keywords extraction and target domain name finder,” Decis. Support Syst., vol. 88, pp. 18–27, 2016, doi: 10.1016/j.dss.2016.05.005.
G. Varshney, M. Misra, and P. Atrey, “Browshing a new way of phishing using a malicious browser extension,” 2017, doi: 10.1109/IPACT.2017.8245147.
M. Babagoli, M. P. Aghababa, and V. Solouk, “Heuristic nonlinear regression strategy for detecting phishing websites,” Soft Comput., vol. 23, pp. 4315–4327, 2019, doi: 10.1007/s00500-018-3084-2.
I. Tyagi, J. Shad, S. Sharma, S. Gaur, and G. Kaur, “A Novel Machine Learning Approach to Detect Phishing Websites,” 2018 5th Int. Conf. Signal Process. Integr. Networks, SPIN 2018, pp. 425–430, 2018, doi: 10.1109/SPIN.2018.8474040.
J. Moedjahedy, H. Zein, I. B, E. Tongalu, K. Kusrini, and M. S. Mustafa, “Analisis Seleksi Tingkat Kecocokan Gambar pada MDID Multimedia Database Dengan Menggunakan Metode ImageDNA,” CogITo Smart J., 2020, doi: 10.31154/cogito.v6i1.223.50-59.
K. L. Chiew, C. L. Tan, K. S. Wong, K. S. C. Yong, and W. K. Tiong, “A new hybrid ensemble feature selection framework for machine learning-based phishing detection system,” Inf. Sci. (Ny)., vol. 484, pp. 153–166, 2019, doi: 10.1016/j.ins.2019.01.064.
I. Salihovicd, H. Serdavic, and J. Kevric, “The Role of Feature Selection in Machine Learning for Detection of Spam and Phishing Attacks,” in International Symposium on Innovative and Interdisciplinary Applications of Advanced Technologies (IAT), 2019, vol. 3, pp. 476–483, doi: 10.1007/978-3-030-02577-9.
R. S. Rao and A. R. Pais, “Detection of phishing websites using an efficient feature-based machine learning framework,” Neural Comput. Appl., vol. 31, no. 8, pp. 3851–3873, 2019, doi: 10.1007/s00521-017-3305-0.
J. Mao et al., “Detecting Phishing Websites via Aggregation Analysis of Page Layouts,” Procedia Comput. Sci., vol. 129, pp. 224–230, 2018, doi: 10.1016/j.procs.2018.03.053.
D. Dua and C. Graff, UCI Machine Learning Repository [http://archive.ics.uci.edu/ml]. 2019.
F. J. Gravetter and L. B. Wallnau, Essentials of Statistics for the Behavioral Science, Sixth. Belmon: Thomson Learning, 2008.
U. Nehmzow, Scientific methods in mobile robotics: Quantitative analysis of agent behaviour. Springer Verlag, 2006.
W. Lowie and B. Seton, Essential Statistics for Applied Linguistics. Red Globe Press, 2013.
D. N. Reshef et al., “Detecting novel associations in large data sets,” Science (80-. )., vol. 334, no. 6062, pp. 1518–1524, 2011, doi: 10.1126/science.1205438.
Y. A. Reshef, D. N. Reshef, H. K. Finucane, P. C. Sabeti, and M. Mitzenmacher, “Measuring dependence powerfully and equitably,” J. Mach. Learn. Res., vol. 17, pp. 1–63, 2016.
D. Albanese, S. Riccadonna, C. Donati, and P. Franceschi, “A practical tool for maximal information coefficient analysis,” Gigascience, vol. 7, no. 4, pp. 1–8, 2018, doi: 10.1093/gigascience/giy032.
DOI: http://dx.doi.org/10.22303/csrid.12.2.2020.107-116
Refbacks
- Saat ini tidak ada refbacks.
##submission.copyrightStatement##
##submission.license.cc.by4.footer##
INDEXED BY:
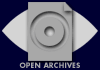

This work is licensed under a Creative Commons Attribution 4.0 International License.
CSRID Journal Editor's Office:
Universitas Potensi Utama. Jl. K.L. Yos Sudarso Km 6,5 No.3-A Telp. (061) 6640525 Ext. 214 Tanjung Mulia Medan 20241
Komentar di artikel ini
oleh karik 1 (2020-09-11)
oleh romanwell romanwell (2020-09-11)
oleh hjhjhrtgre hjhjh (2020-09-13)
oleh milliya well (2020-09-15)
oleh julian98 julian98 (2020-09-18)
oleh jing markas 4d hago (2020-10-01)
oleh Anon Anon (2020-10-04)
oleh rem bd22 (2020-10-05)
oleh bin z (2020-10-06)
oleh menawell well (2020-10-19)
oleh emi ly (2020-10-28)
oleh silicat silicat (2020-11-10)
oleh su beo (2020-11-17)
oleh autumno autumno (2020-11-30)
oleh lo vell (2020-12-10)
oleh hai vuong (2020-12-12)
oleh ken dy (2020-12-30)
oleh mert34 mert mert (2021-01-05)
oleh mert34 mert mert (2021-01-05)
oleh jack jeless (2021-01-06)
oleh mert34 mert mert (2021-01-12)