Analisa Sentimen Dengan Korpus Sentiment140 Menggunakan Classifier Support Vector Machine RBF
Sari
Kata Kunci
Teks Lengkap:
PDFReferensi
M. D. Landry, L. Geddes, A. P. Moseman, J. P. Lefler, S. R. Raman, and J. van Wijchen, “Early Reflection on the Global Impact of COVID19, and Implications for Physiotherapy,” Physiotherapy, 2020.
J. A. Lewnard and N. C. Lo, “Scientific and ethical basis for social-distancing interventions against COVID-19,” Lancet Infect. Dis., vol. 3099, no. 20, pp. 2019–2020, 2020.
R. M. Anderson, H. Heesterbeek, D. Klinkenberg, and T. D. Hollingsworth, “How will country-based mitigation measures influence the course of the COVID-19 epidemic?,” Lancet, vol. 395, no. 10228, pp. 931–934, Mar. 2020.
A. Shelar and C. Y. Huang, “Sentiment analysis of twitter data,” Proc. - 2018 Int. Conf. Comput. Sci. Comput. Intell. CSCI 2018, pp. 1301–1302, 2018.
B. Gokulakrishnan, P. Priyanthan, T. Ragavan, N. Prasath, and A. Perera, “Opinion mining and sentiment analysis on a Twitter data stream,” Int. Conf. Adv. ICT Emerg. Reg. ICTer 2012 - Conf. Proc., pp. 182–188, 2012.
M. Grandjean, “A social network analysis of Twitter: Mapping the digital humanities community,” Cogent Arts Humanit., vol. 3, no. 1, pp. 1–14, 2016.
F. Abel, Q. Gao, G. J. Houben, and K. Tao, “Analyzing user modeling on Twitter for personalized news recommendations,” Lect. Notes Comput. Sci. (including Subser. Lect. Notes Artif. Intell. Lect. Notes Bioinformatics), vol. 6787 LNCS, pp. 1–12, 2011.
M. Darwich, S. A. Mohd Noah, N. Omar, and N. A. Osman, “Corpus-Based Techniques for Sentiment Lexicon Generation: A Review,” J. Digit. Inf. Manag., vol. 17, no. 5, p. 296, Oct. 2019.
R. M. A. Baracho, M. P. Bax, L. G. F. Ferreira, and G. C. Silva, “Sentiment analysis in social networks,” in 5th International Multi-Conference on Complexity, Informatics and Cybernetics, IMCIC 2014 and 5th International Conference on Society and Information Technologies, ICSIT 2014 - Proceedings, 2014, pp. 20–25.
H. Shirdastian, M. Laroche, and M. O. Richard, “Using big data analytics to study brand authenticity sentiments: The case of Starbucks on Twitter,” Int. J. Inf. Manage., vol. 48, no. April, pp. 291–307, 2019.
P. Ducange, M. Fazzolari, M. Petrocchi, and M. Vecchio, “An effective Decision Support System for social media listening based on cross-source sentiment analysis models,” Eng. Appl. Artif. Intell., vol. 78, no. May 2018, pp. 71–85, 2019.
M. Awad and R. Khanna, Efficient Learning Machines. Berkeley, CA: Apress, 2015.
J. Mueller and L. Massaron, Machine learning for dummies. 2016.
H. J. G. Palacios, R. A. J. Toledo, G. A. H. Pantoja, and Á. A. M. Navarro, “A comparative between CRISP-DM and SEMMA through the construction of a MODIS repository for studies of land use and cover change,” Adv. Sci. Technol. Eng. Syst. J., vol. 2, no. 3, pp. 598–604, Jun. 2017.
J. Silge and D. Robinson, Text Mining with R Text Mining with R Revision History for the First Edition. 2017.
V. Vyas and V. Uma, “An Extensive study of Sentiment Analysis tools and Binary Classification of tweets using Rapid Miner,” Procedia Comput. Sci., vol. 125, pp. 329–335, 2018.
Y. Al Amrani, M. Lazaar, and K. E. El Kadirp, “Random forest and support vector machine based hybrid approach to sentiment analysis,” Procedia Comput. Sci., vol. 127, pp. 511–520, 2018.
Ankit and N. Saleena, “An Ensemble Classification System for Twitter Sentiment Analysis,” Procedia Comput. Sci., vol. 132, no. Iccids, pp. 937–946, 2018.
J. T. Wixted, L. Mickes, S. A. Wetmore, S. D. Gronlund, and J. S. Neuschatz, “ROC Analysis in Theory and Practice,” J. Appl. Res. Mem. Cogn., vol. 6, no. 3, pp. 343–351, 2017.
DOI: http://dx.doi.org/10.22303/csrid.12.2.2020.89-97
Refbacks
- Saat ini tidak ada refbacks.
##submission.copyrightStatement##
##submission.license.cc.by4.footer##
INDEXED BY:
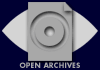

This work is licensed under a Creative Commons Attribution 4.0 International License.
CSRID Journal Editor's Office:
Universitas Potensi Utama. Jl. K.L. Yos Sudarso Km 6,5 No.3-A Telp. (061) 6640525 Ext. 214 Tanjung Mulia Medan 20241
Komentar di artikel ini
oleh Pabrik Jam (2020-08-19)
oleh Barokah Catering (2020-08-22)
oleh joan joan davis davis (2020-09-01)
oleh dorcas smith smith (2020-09-02)
oleh Lisandra Lisandra Ferreira Silva (2020-09-09)
oleh Lisandra Lisandra Ferreira Silva (2020-09-09)
oleh rem bd99 (2020-09-14)
oleh Souvenir Jam (2020-09-22)
oleh Hotmail Entrar (2020-09-25)
oleh Hotmail Entrar (2020-09-25)
oleh wmap WELL (2020-09-25)
oleh Anon Anon (2020-10-10)
oleh Hotmail Entrar (2020-10-14)
oleh Mr thai nguyen duong (2020-10-14)
oleh ricardo oliveira (2020-10-20)
oleh shen paker (2020-11-20)
oleh uy tin6996 (2020-11-26)
oleh grace edens (2020-11-28)
oleh grace edens (2020-11-28)
oleh grace edens (2020-11-28)
oleh Yohana Safitri (2020-12-04)
oleh skandal situs slot online resmi (2020-12-05)
oleh grace edens (2020-12-05)
oleh loc mb11 (2020-12-08)
oleh Mr buy khoem (2020-12-18)
oleh grace edens (2020-12-31)
oleh grace edens (2020-12-31)
oleh grace edens (2020-12-31)
oleh grace edens (2020-12-31)
oleh grace edens (2021-01-08)
oleh grace edens (2021-01-08)
oleh grace edens (2021-01-08)